† Corresponding author. E-mail:
Various soft materials share some common features, such as significant entropic effect, large fluctuations, sensitivity to thermodynamic conditions, and mesoscopic characteristic spatial and temporal scales. However, no quantitative definitions have yet been provided for soft matter, and the intrinsic mechanisms leading to their common features are unclear. In this work, from the viewpoint of statistical mechanics, we show that soft matter works in the vicinity of a specific thermodynamic state named moderate point, at which entropy and enthalpy contributions among substates along a certain order parameter are well balanced or have a minimal difference. Around the moderate point, the order parameter fluctuation, the associated response function, and the spatial correlation length maximize, which explains the large fluctuation, the sensitivity to thermodynamic conditions, and mesoscopic spatial and temporal scales of soft matter, respectively. Possible applications to switching chemical bonds or allosteric biomachines determining their best working temperatures are also briefly discussed.
Named by Pierre–Gilles de Gennes in his Nobel Prize lecture,[1,2] “soft matter” vaguely refers to a wide spectrum of condensed materials, ranging from polymers, complex liquids, liquid crystals to foams, colloids, gels, granular materials, and biological materials. Various soft materials share some common features, such as significant entropic effect, large fluctuations, sensitivity to thermodynamic conditions (particularly a weak force can induce a large transformation), mesoscopic characteristic spatial and temporal scales, and the ability of self-assembly.[3] Despite successes in some individual fields, such as polymers[4] and liquid crystals,[5] no general quantitative definitions have yet been provided for soft matter, and the intrinsic mechanisms leading to their common features are unclear.
Regarding the subtle balance between entropy and enthalpy contributions in soft matter, the term “entropy–enthalpy compensation” has been frequently carried out since 1950s, aiming to quantitatively describe the unique characteristics of soft matter (see, e.g., Refs. [6–14]). Initially, entropy–enthalpy compensation was referred to the phenomenon that a change in thermodynamic condition leads to almost identical changes in entropy and enthalpy, for the Helmholtz free energy
![]() | (1) |
![]() | (2) |
The physical interpretation of this form is that the free energy cost
The current forms of the above two terms, however, neither reveal the statistical physics essence of soft matter nor provide a systematic explanation of the common features soft materials exhibit. The problem may reside in the fact that different thermodynamic states are compared to looking for the balance or compensation between thermodynamic entropy and enthalpy. Instead, in this paper, we will show that the balanced condition is satisfied in a single thermodynamic state, whose substates along an order parameter (or more general a reaction coordinate) have various ratios of entropy and enthalpy contributions rather than thermodynamic variables of entropy or enthalpy. By looking into the substates of the free energy landscape along a predefined order parameter, we will define soft matter as the materials working in the vicinity of a moderate point, at which entropy and enthalpy contributions among substates are well balanced (or have a minimal difference when the exact balanced condition cannot be satisfied). This (quasi-)balanced condition leads to the maximization of the order parameter fluctuation around the moderate point, which explains the intrinsic large fluctuation of soft matter. The associated response function also maximizes around the moderate point, which explains the sensitivity of soft materials to thermodynamic conditions. Their mesoscopic temporal and spatial scales may be explained by the maximization of the finite spatial correlation length around the moderate point. For convenience, we will call our theory the moderation theory.
From now on, we assume that by default the system is in the canonical ensemble, and “enthalpy” actually refers to the system total energy. The essential idea of the moderation theory is that the subtle balance between the entropy and enthalpy contributions of soft matter should be reflected by the probability distribution (or equivalently the free energy landscape) of all substates of a thermodynamic state (a state point in the phase space). With an appropriately defined order parameter, a thermodynamic system with very high dimensions (e.g., a system with N particles in the 3-D space has 6N degrees of freedom) is projected into a reduced phase space spanned by the order parameter. Note that here “substates” are microstates in the reduced phase space, not in the original full-dimensional space. For simplicity, in this paper, only the case with one order parameter h is considered, along with a generalized field B conjugated with h. Nevertheless, it should be straightforward to generalize the theory to the cases with multiple order parameters.
The Helmholtz free energy of a thermodynamic state point is
![]() | (3) |


![]() | (4) |



![]() | (5) |
Note that
Without considering the generalized field B, for the simplest case of a classical statistical system with only two substates: entropy-dominated state 1 and enthalpy-dominated state 2 after projecting into the phase space spanned by an order parameter h, if the system energies of the two substates are U1 and U2 with
![]() | (6) |
![]() | (7) |
If the generalized field B is considered with
![]() | (8) |






In Appendix
When the substates are continuous, it is unobvious what kind of probability distributions have balanced entropy and enthalpy contributions. Our strategy is dividing the whole probability distribution into two parts by the ensemble average (expected value) of the order parameter
![]() | (9) |



![]() | (10) |


As shown in Appendix
![]() | (11) |

In the linear response regime, the system Hamiltonian under a generalized field B can be written as
![]() | (12) |
![]() | (13) |
Since the total free energy is
![]() | (14) |
The response function corresponding to B is
![]() | (15) |
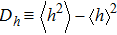
According to Eq. (
The characteristic spatial scale can be quantified by the spatial correlation length. In the linear response regime, the spatial correlation length can be related to the response function as
![]() | (16) |


![]() | (17) |
In Appendix
The validity of the moderation theory was examined with a simplified polypeptide aggregation model. Previously, we simulated by coarse-grained molecular dynamics the aggregation behaviour of polyglutamine molecules in aqueous solution.[23,24] We found that, in equilibrium, the instantaneous configurations of polyglutamine molecules fluctuate from distributing almost uniformly to aggregating very tightly, and the degree of aggregation decreases monotonically with temperature but first increases and then decreases with concentration. Based on the simulation data, we then projected this high-dimensional system into a one-dimensional phase space spanned by the so-called heterogeneity order parameter (HOP).[25] characterizing the degree of aggregation, defined as
![]() | (18) |




![]() | (19) |
Since the momentum space is independent of the configurational space,
For the system with 27 32-residue polyglutamines, by fitting the simulation data, we determine the density of states
![]() | (20) |
![]() | (21) |




![]() | (22) |
The generalized field associated with h is
![]() | (23) |
![]() | (24) |
In this model, the fluctuation of the order parameter is a function of both temperature and concentration
The probability density distributions
![]() | Fig. 2. Probability density distributions at (a) ![]() ![]() |
For a fixed temperature, varying L can also change the balance between entropy and enthalpy. The distributions at an arbitrarily selected
The response function χ corresponding to h has been calculated according to Eq. (
The moderation theory should be quite general and should be able to be applied to most (if not all) soft materials. A possible application of the two-substate model moderation theory could be the case of chemical bond breaking and reformation. If all microsates of a chemical bond can be grouped into only two substates independent of temperature: the enthalpy-dominated connected state and the entropy-dominated broken state, then at the moderate temperature given by Eq. (
Another possible application is the determination of the best working temperature of biochannels. A biochannel works the best when the difference between the open state and the close state, modulated by a binary variable (e.g., binding and unbinding of an ion on a specific binding site)
![]() | (25) |
If the system energy U linearly depends on h, the maximization requirement
![]() | (26) |
Alternatively, integrating the first part of Eq. (
![]() | (27) |
Along with the second part of Eq. (
![]() | (28) |
Either Eq. (
In summary, we developed the moderation theory for soft matter by defining the moderate point of a statistical system at which the entropy and enthalpy contributions among substates along an order parameter perfectly balance each other or have a minimal possible difference. The order parameter fluctuation maximizes around the moderate point. Soft materials work in the vicinity of the moderate point, which explains their sensitivity to thermodynamic condition changes via the relation between the order parameter fluctuation and the associated response function. The mesoscopic characteristic spatial and temporal scales of soft matter are also explained by the maximization of the finite spatial correlation length at the moderate point. The moderation theory was validated by the simplified statistical model for polyglutamine aggregation, and its possible applications to switching chemical bonds and biochannels were also briefly discussed. The moderation theory is anticipated to form the basis of the theoretical framework providing a quantitative definition for soft matter.
An important feature of the moderation theory is that the moderate point heavily depends on the order parameter because a specific choice of an order parameter reflects the perspective of the study for a given soft material. In addition, it is worth emphasizing that, the maximization of the response function is not always exactly at the moderate point, and there are no distinct boundaries for the entropy and enthalpy difference dividing the “soft” and “non-soft” regions of the system, reflecting the “softness” feature of soft matter.
More questions related to the moderation theory for soft matter are open for investigation. Figure
[1] | |
[2] | |
[3] | |
[4] | |
[5] | |
[6] | |
[7] | |
[8] | |
[9] | |
[10] | |
[11] | |
[12] | |
[13] | |
[14] | |
[15] | |
[16] | |
[17] | |
[18] | |
[19] | |
[20] | |
[21] | |
[22] | |
[23] | |
[24] | |
[25] | |
[26] | |
[27] | |
[28] | |
[29] | |
[30] | |
[31] | |
[32] |